Interview by Daniela Silva
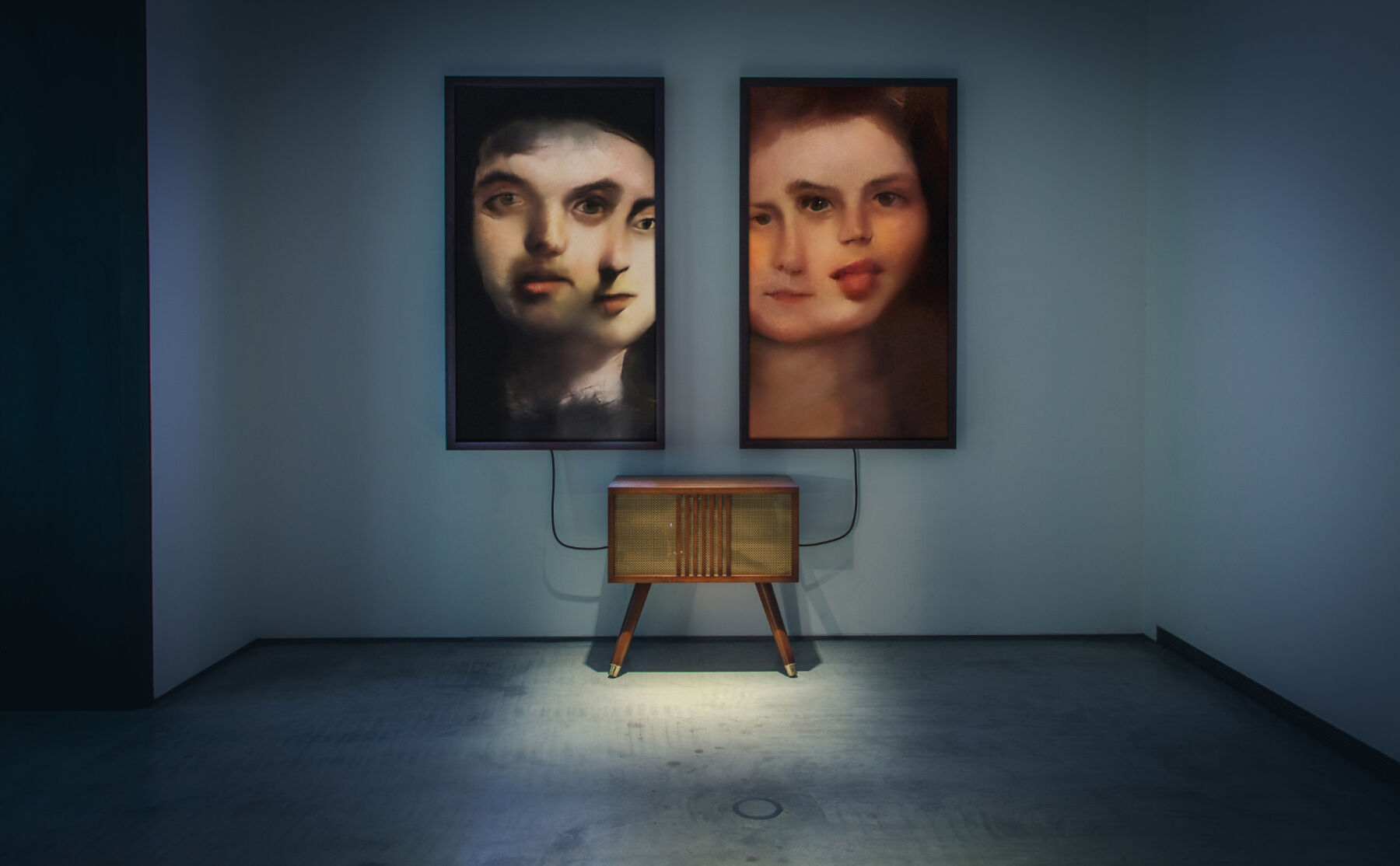
Mario Klingemann is a pioneering figure in the intersection of technology and creativity, renowned for his innovative use of neural networks in art. Deeply immersed in the AI art community, Klingemann offers invaluable insights into trends such as data poisoning, where AI algorithms are deliberately corrupted to challenge traditional notions of creativity and authorship. His expertise sheds light on the ethical implications and technical challenges of incorporating AI into artistic practices. His journey began in the mid-1980s when he taught himself programming, which sparked his fascination with the endless possibilities of digital bitmaps. He envisioned a digital universe where any conceivable image, text, or formula could exist. However, he quickly realised that brute-force methods needed to be revised to navigate this vast combinatorial space. This quest for a more intelligent approach to image generation has driven his artistic practice for nearly four decades.
Klingemann’s early work in generative and algorithmic art was motivated by a desire to create aesthetically appealing images with intentional qualities. The need to manually sift through outputs led him to a crucial question: How can machines be taught to recognise and optimise for “interestingness”? This inquiry naturally led him to artificial intelligence, especially as the profound learning revolution began. He sees AI not as a collaborator but as a complex instrument, like a piano, through which he expresses his artistic vision. He emphasises human agency in the creative process, using AI as a medium rather than a creator. The unpredictability of AI outputs often leads to surprising results, which Klingemann views as a byproduct of the interplay between the model’s training data, architecture, and inputs.
A key aspect of his work is his engagement with data poisoning, a practice driven by fears and frustrations within the art community. Artists use it to protect their creative identity by corrupting AI training data. Klingemann believes this trend is a reaction to the disruption caused by AI, particularly in digital art. He argues that protective measures are futile as AI continues evolving using synthetic data. Instead, he suggests that artists embrace visibility within AI systems, ensuring their work is accurately recognised and categorised to future-proof their artistic identity. The ethical and technical challenges of creating art with neural networks are significant too. Klingemann often works with open-source models, which offer the freedom to experiment despite their limitations. The black-box nature of these networks requires a process of trial and error, where artistic intuition and persistence are crucial. Maintaining a unique creative voice in a field where tools can overshadow the artist is a delicate balance that Mario navigates skillfully.
Klingemann’s contributions to AI art are profound explorations of what it means to create in the digital age. His work challenges us to reconsider creativity’s boundaries, the ethical implications of technology, and the evolving role of the artist, providing a roadmap for navigating this complex and exciting landscape.
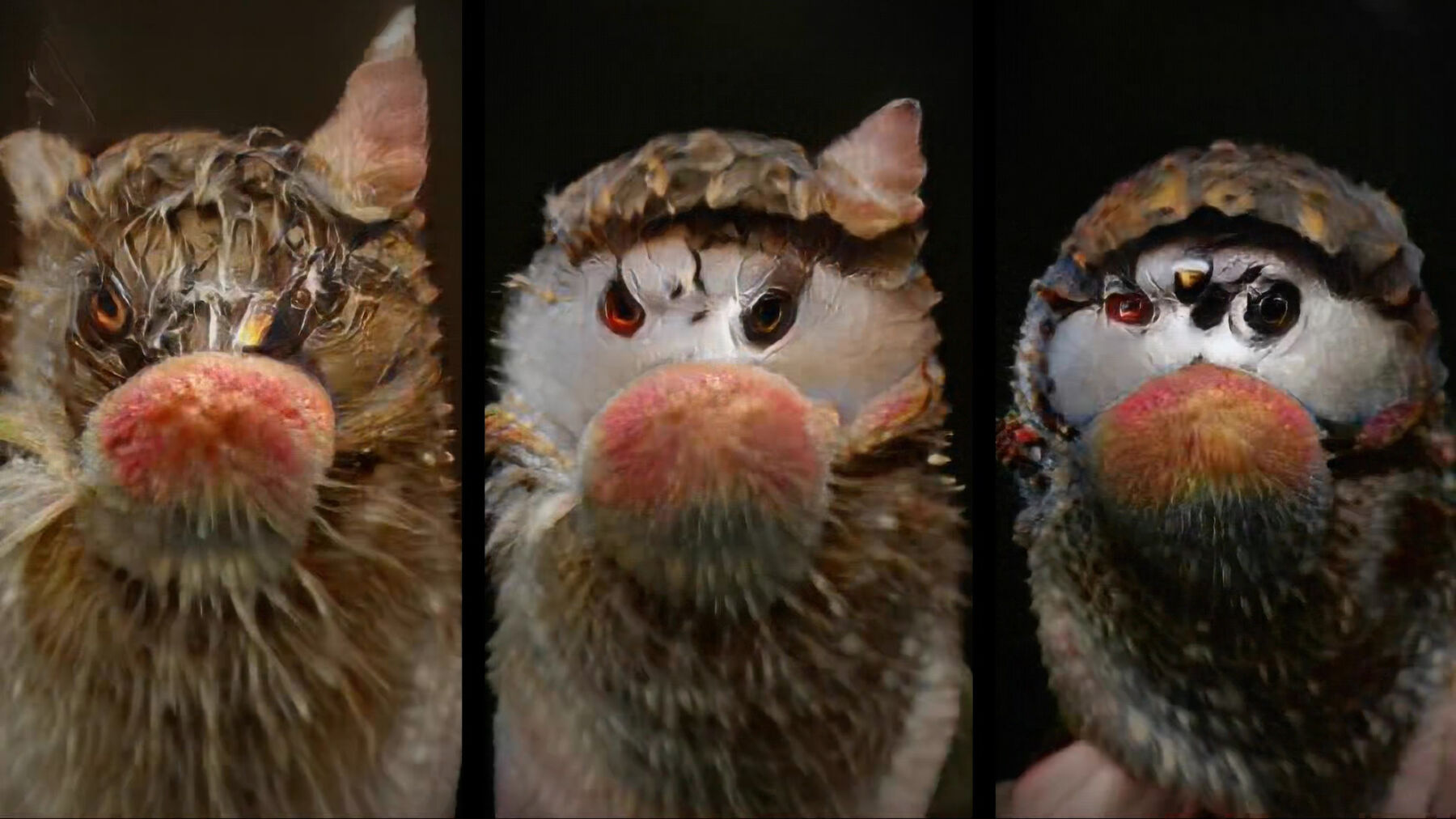
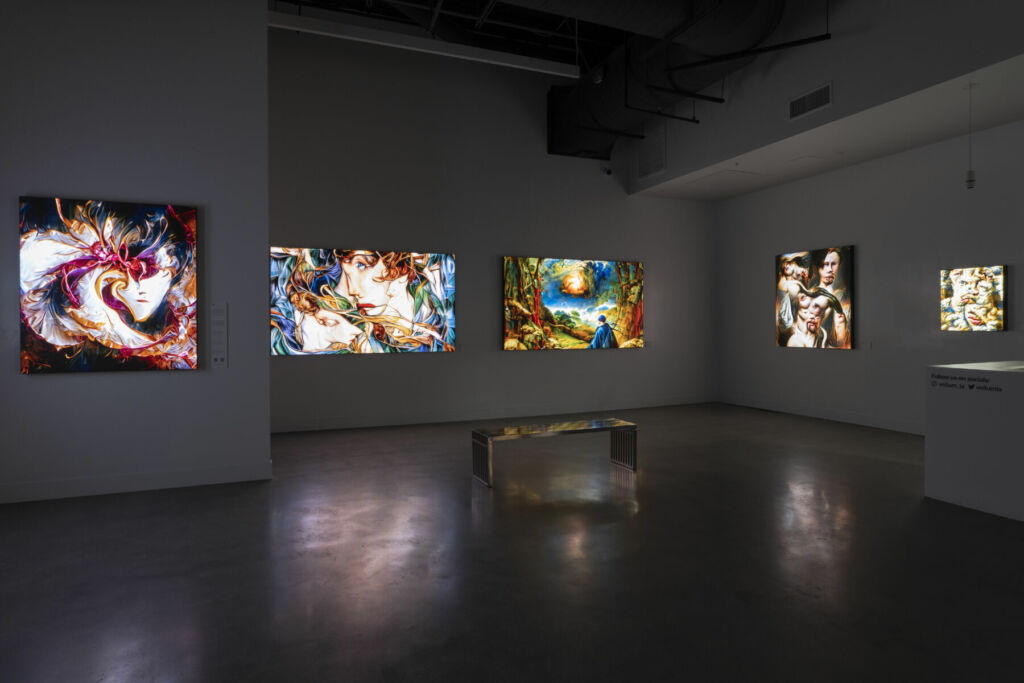
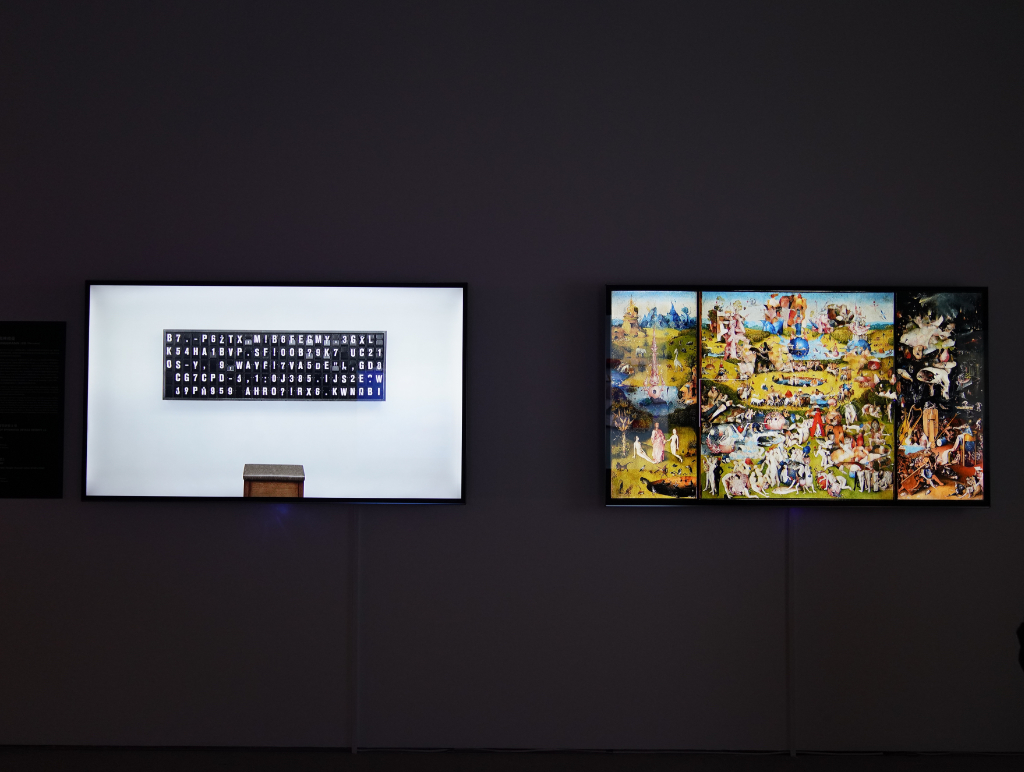
Can you share what initially drew you to the intersection of AI and art and how your background influenced your journey as a pioneer in using neural networks for artistic purposes?
Employing AI in my practice was an almost inevitable step in my artistic evolution. It resulted from the ideas and questions I had become increasingly focused on for nearly 25 years, starting in the mid-1980s when I taught myself programming. My journey started in my early teenage years with the realisation that from a mathematical standpoint, a digital bitmap can, at least in theory, show me any image that has ever been created, any image that will ever be created and any image that might never be created (by humans) in case some cosmic or man-made catastrophe ends our civilisation. Of course, these images also include depictions of texts, meaning that as an additional bonus, they would include any book, source code, or mathematical formula possible. What an exciting outlook – all I had to do was to iterate through all the pixel permutations, sit back and pick the best parts.
So I wrote the simple program necessary and waited. After half an hour of looking at a black screen on which a few white pixels flickering in the top left corner, I had to realise that combinatorics were not on my side and that with this brute-force approach, I might be lucky if those pixels would at least extend to the other end of the screen during my lifetime. It also dawned on me that I could not watch that screen 24 hours a day, so how could I make sure that if an interesting or at least promising image came by, I would notice it? It was also clear that 99.999% of the time, the images I would see would be meaningless noise or minor variations of similar ones.
This hopeless approach needed a more intelligent way to separate the signal from the noise. Improving this method has been one of the driving forces of my artistic practice over the past almost 40 years. Of course, my questions and methods evolved with my growing experience and as with any good journey, it’s not really about the destination but the friends you make while you are on the road.
A few of the stepping-stone questions along the way were: What ways are there to create images automatically that are not just noise but have some aesthetic appeal or appear to have intentional qualities in form or composition? This brought me into generative/algorithmic art, allowing me to explore small subsections of the image-possibility space. While this approach does have a much better ratio when it comes to time spent on the process versus the number of exciting results one gets out of it, it still required me to judge every single output and adjust the parameters of the system to get it to give me more of what I was looking for or found appealing and less of the redundant or unappealing material.
So the next logical question was: how can I make the machine see what I see, make judgments and make informed changes to the process to optimise the outcome autonomously? This opened up an entirely new can of worms, which could be summarised by “What is interestingness?” and “Why is this art and that not?” which I approached from a less philosophical but somewhat analytical standpoint. I learned then that any meaningful image comes with a cultural subtext that is not necessarily encoded in the pixels themselves but emerges from the individual personal experience and the accumulated cultural context that civilisation carries.
This was the moment when AI became a necessity. Fortunately, I only had to wait a few more years until the deep learning revolution started, at first bringing classifiers that were able to capture entire concepts or even deeper semantics and, a bit later, models that were able to reverse the process and allow me to create images that tried to visualise this “understanding” they had gained about our world through vast amounts of training data.
Most eye-opening for me was eventually understanding the concept of multidimensional latent spaces, which – spoken in a simplified manner – resemble the innermost universe of these models and their way of rearranging and ordering the training information they are given in a way that optimises their ability to predict, classify or generate once the training is finished and which as a side effect reveal inherent patterns in the data, in particular those that we might not have noticed or others for which we might not even have words.
How do you approach the creative process when working with AI? Do you see the machine as a collaborator, a tool, or something else?
When working with AI, the creative process is a fascinating dance between human intention and machine capability. Many years ago, I described AI as not just a tool but an instrument. This analogy still holds true for me today.
Think of AI as a complex scientific “periscope”—imagine a combination of a microscope and a telescope, only that it allows us to look into latent spaces. It augments our perception, allowing us to see the world at previously inaccessible scales or levels. But it’s more than just a way of seeing—it’s also a means of expression. In this sense, AI is like a piano. It’s a complex instrument that, when performed skillfully, gives us new ways of expression that our body alone cannot produce. Just as a pianist can create intricate melodies and harmonies beyond what human vocals could achieve, AI allows us to create visual and conceptual compositions that stretch far beyond what our hands alone could craft.
But here’s the crucial point: we don’t credit a piano for a musical performance. The credit goes to the person who performs on it, who brings their skill, emotion, and intention to the keys. The same holds for creating with AI. The machine doesn’t create the art—I do. AI is the instrument I play, the medium through which I express my ideas and explore new creative territories.
This perspective shapes my entire approach to the creative process. I don’t see the machine as a collaborator in the traditional sense. It needs to be an equal partner in the creative dialogue. Instead, it’s a sophisticated system that I interact with, probe, and manipulate to achieve my artistic vision. The process often starts with a question or a concept I want to explore. Then, it becomes a matter of figuring out which AI “instrument” might best suit that exploration and how I might need to modify or fine-tune it to get closer to what I envision. Sometimes, this means combining multiple models or techniques or even developing entirely new approaches.
This process always has an element of unpredictability, which I find exhilarating. The AI might produce outputs that surprise me, leading to new ideas or directions I hadn’t initially considered. But these surprises aren’t the AI being “creative” – they result from the complex interactions between the model’s training data, architecture, and the inputs I provide. My role is to recognise these outputs’ potential, shape and refine them, and contextualise them within my broader artistic vision.
Ultimately, working with AI is about expanding the boundaries of what’s possible in art. It’s about using these new instruments to explore questions about perception, creativity, and the nature of intelligence itself. The machine isn’t my collaborator or tool – it’s my instrument, medium, and means of pushing the envelope of artistic expression in the digital age.
Could you elaborate on the inspiration and the technical process behind creating Neural Decay? How did you balance the aesthetic elements with the underlying AI algorithms to achieve the desired effect?
I have difficulties naming specific inspirations for my works. Most of the time, the art you see is more of a manifested snapshot of my current experimentation or interest. They are like proofs of work necessary to justify one’s role as an artist. If possible, I’d fully indulge in the process of creation and exploration and never release any “finished” work into the world. In the case of Neural Decay, I embraced my long-time love for “beautiful decay” that manifests itself in the form of rusted surfaces, peeling paint, or withered organic structures. I have been collecting interesting surface details since I had my first camera as a teenager. I captured some of the essential features I appreciate in this work by training a custom model on a small selection.
The history of the AI models over the past 10+ years has been chiefly the history of transforming some A into some B, particularly the growing list of what nature A and B can be. Early on, A was mostly just images and B numbers: classifier models that, for example, allowed the detect the likelihood that there is a dog or a cat shown in an image. Then, a bit later, B could also be images that opened up the world of image-to-image transformations. A few years later, A could also be texts that started the text-to-image prompting era that most people today associate with AI art. Today, A and B can be almost anything, and our models can be universal transformers that transform one manifestation of information into another.
When I created Neural Decay, image-to-image transformations had just been made accessible via models named “pix-to-pix” and “CycleGAN,” and I was experimenting with their possibilities. Here, I am employing a model I had tried to train to transform human faces into doll faces, which involved changing their facial proportions and increasing their eyes.
While the model seemingly did “understand” the goal, it never became perfect and introduced artefacts and erroneous mutations. I spent a lot of time trying to fix these mistakes and make the outcome more “perfect” by retraining the model or altering its internal architecture—only later did I realise that precisely those imperfections and “mistranslations” made the outcome interesting and unique.
Data poisoning, where AI algorithms are intentionally corrupted, is an emerging trend in the AI art community. What are your thoughts on this practice, and how do you believe it impacts the notions of creativity and authenticity?
Before going into data poisoning, let me address the broader context driving these practices. I fully understand the fears of traditional artists facing changes threatening their livelihood. Many of my friends in the conventional art world are directly affected, with once-common funding sources like agency work or editorial commissions drying up. Unfortunately, I don’t have consoling words or advice for them. This trend is unlikely to reverse – it will likely worsen.
This is simply how technology constantly changes our ways of working and consuming. The only way to stay afloat is adaptability. Sadly, most of those now threatened by AI would only be doing what they’re doing with technological progress, handing them their tools and opening new channels to find audiences. I’m referring to digital artists, who are among the hardest hit by AI. Over the past 30 years, digital tools made illustration, graphic design, and photography using traditional analog media an expensive luxury for high budgets and less time-pressured projects. Unfortunately, for day-to-day “consumable” work, the days of those still working in this manner are numbered, much like typesetters were replaced by desktop publishing.
Now, to address data poisoning specifically, these attempts to protect one’s creations are driven by fear and anger. Fear of losing one’s livelihood or unique identity and anger that years spent perfecting one’s craft and carving out a niche doesn’t pay off as the world changes. Unfortunately, fear and anger are poor advisors. They drive people into the hands of charlatans and snake oil vendors promising ways to protect their labour – in this case, data poisoning tools. These are merely band-aids that might work briefly, but just as there will likely never be a remedy for the common cold as viruses adapt, humans will always find new ways to solve challenges.
Another issue is that these attempts should be coming earlier. We’ve reached a point where “real” data is often no longer necessary to improve current models. They can self-improve by creating synthetic data. This brings us to a critical factor in making a living as an artist: recognizability and staying on people’s minds. An artist’s goal should be to reach a point where people say, “This reminds me of X’s work.” Of course, you want them to commission you when they have a project fitting your style rather than having someone cheaper execute it based on a Pinterest board assembled from your work (a common practice even before AI).
The thing is that once you have reached that point, at least big brands that have a name to lose cannot afford NOT to have you do work for them that everyone will associate with you since if they try to, they will face a social media shitstorm that costs more to contain than having commissioned you in the first place. Imagine Nike doing a campaign “in the style of Greg Rutkowski.” It would take seconds after their first tweet before their threads start filling up with angry comments, and the press would have a feast by exposing their rip-off in the days to come.
I must admit that I had never heard of Greg Rutkowski before MidJourney.
Pre-AI, one way to ensure recognition was to get your name and work out in the world by any means necessary, providing you were found on Google when people searched for someone with your skills. With AI increasingly replacing Wikipedia and Google for this kind of research, trying to prevent these models from knowing about you is the most counterproductive thing you can do. In this new landscape, visibility within AI systems becomes crucial. The very tools that some artists fear could become their most powerful allies in maintaining relevance and securing work. By ensuring your work is recognised and categorised by AI, you’re future-proofing your artistic identity.
This shift requires a fundamental change in how we think about exposure and copyright. Instead of trying to hide or protect our work from AI, we should consider how to best present it to these systems. The challenge becomes not how to prevent AI from learning about your work but how to ensure it learns about it accurately and comprehensively. I’ve noticed increased voices demanding expanded copyright protections and more upload scanning and control. However, this won’t have the consequences and effects those asking for it hope for. Many of them might be the ones most negatively affected.
First, the irony: a system checking whether a style or concept is already “owned” by someone could only be realized with AI. However, measuring the similarity between two things is never an exact science. Take images: even if two are considered sufficiently similar, there are plenty of factors that can’t be derived from the image alone, and publishing the second one would be entirely within the rights of the person doing it.
Those promoting such measures envision global institutions tracking everything everyone creates, collecting fees, sending DMCA notices, or taking down content. Imagine the bureaucratic apparatus this would require… Yes, this works well for large publishers and companies owning lots of content. However, individual artists won’t profit in any way that makes a dent in their wallet – what’s left after everyone else takes their percentages or administration fees will be negligible. And, of course, among the loudest who are trying to establish these systems, some are trying to set up these middle-men entities that “take care” of artists since they could become very lucrative – for those who operate them.
Simultaneously, in this scenario, when artists try to upload their original work, they might face warning messages that their work cannot be uploaded since it looks too similar to someone else’s (or, as it has already happened, “like AI”). But here’s a 5-page form to dispute this (processing time: approximately ten weeks – priority processing available for just $20). We already see how well this works in music, where payouts for anyone but the top 1% of stars are a joke, and unjustified takedown notices for presumed copyright infringement on platforms like YouTube are legion.
This approach to copyright protection is shortsighted and potentially harmful to the creators it claims to protect. It risks creating a system where large corporations and institutions have even more control over creative expression while individual artists face increasing barriers to sharing their work.
I have no issue with using models that have been trained on material from any source – with consent or without. And you might hate me for that, but I am a “civilisation maximalist” – we are the only known species that we know managed to convert sunlight into information (which, of course, includes art) and can pass it on to future generations so they do not have to reinvent the wheel. And AI is the next step in that evolution. Creating art is the highest luxury our species can afford to spend their time on since it is unnecessary for survival, and there is no natural right that says you also have to be able to make a living.
For me, responsibility begins not with what I create with these models but with what I publicly share and declare as “mine.” In that aspect, I would never deliberately try to steal or appropriate the style of someone who is directly affected by that—what would be the point of that, given that I want to find my own voice? And that should be the approach anyone who creates with AI should adopt.
What are some of the most significant technical challenges you face when creating art with neural networks, and how do you overcome them?
The technical challenges of creating art with neural networks are as fascinating as frustrating. Most of the models I worked with weren’t built for artistic purposes, so I’m constantly trying to overcome their limitations or use them in ways their creators never intended. It’s like trying to play a violin with a hammer—possible, but it requires some creative workarounds.
Commercial art models present a different problem. They’re often streamlined and “tamed” to specific popular aesthetics or topics, which usually results in mediocrity. These models churn out images that look like art but need more real depth and originality. It’s as if they’ve been trained to colour within the lines, precisely what I’m trying to avoid. So, I steer clear of them. Open-source models, on the other hand, are my playground of choice. Sure, they might lag a bit behind the closed-source commercial offerings in terms of output quality, but with some effort, you can get pretty close to state-of-the-art results. More importantly, they offer the freedom to experiment, push boundaries, break things, and see what happens.
One of the most intriguing challenges is the black-box nature of these networks. You feed them input, and they spit out output, but what happens in between is often a mystery. It’s like having a conversation with an alien intelligence – you’re always wondering if you’re understanding each other correctly. This leads to a lot of trial and error, a constant process of poking and prodding the model to see how it reacts. However, over time and with more experience, one feels gut about a model’s sweet spots and weak spots.
Overcoming these challenges is about more than just technical know-how. It’s about artistic intuition, persistence, and a willingness to fail repeatedly until something interesting emerges. I spent countless hours fine-tuning models, creating custom datasets, and developing new techniques, trying to bend these digital tools to my will. Still, at the same time, I come halfway over to how the models prefer to be operated, so it’s not just the machine that learns here.
However, the biggest challenge is maintaining a unique artistic voice in a field where the tools can sometimes threaten to overshadow the artist. It’s a delicate balance between leveraging AI’s power and imposing my artistic vision. After all, the goal isn’t just to create visually striking images – it’s to use AI to explore more profound questions about creativity, consciousness, and the nature of art itself. In the end, these technical challenges are what make working with AI so exciting. Each obstacle overcome is a step into uncharted territory, a chance to create something genuinely new. And isn’t that what art is all about?
In a world where AI can generate art, what do you believe is the role of the human artist? How do you see this role evolving in the future?
The role of the human artist in a world where AI can generate art remains crucial. As I’ve maintained for years, humans still create art with AI, not AI creating art autonomously. The increased accessibility of AI tools, however, presents a new set of challenges and considerations for artists. These tools have enabled creative output to be produced with minimal effort at an incredible pace. The result is a never-ending flood of content that threatens to overwhelm our input channels and exceed our capacity for meaningful interaction. We risk becoming numb and tired of looking, increasingly unwilling to pay attention to the torrents of AI-generated material and—as collateral damage, so to say—also the work created by traditional means.
In this context, where creation becomes the new normal, the role of the artist may evolve in an unexpected direction. Rather than contributing more to the ever-growing pile of “content”, artists might need to create less. This seems counterintuitive, but in a world saturated with AI-generated output, the artist might shift towards curation, distillation, and extracting meaning from the noise.
The future artist could function as a digital ecologist, maintaining a delicate balance in the ecosystem of human creativity and machine capability. Our task might be to craft experiences that demand and reward deeper engagement, to create spaces of contemplation amidst the ceaseless flow of AI-generated content.
This doesn’t imply a cessation of innovation or boundary-pushing. Instead, it suggests a more intentional approach to creation. We might focus more on the conceptual aspects of our work, the questions we’re asking and the ideas we’re exploring. The technical challenges of working with AI will persist, but the essence of art might lie increasingly in knowing when and how to employ these powerful tools – and when to refrain from using them. In the future, the value of an artwork might not be measured by its visual complexity or the sophistication of the AI used in its creation. Instead, its worth might be found in its ability to cut through the noise to provoke pause, thought, and emotion in a world constantly bombarded with stimuli.
The role of the human artist in an AI world is to remain fundamentally human. We need to bring our unique perspectives, emotions, and inherent complexity to bear on our work. AI should undoubtedly be used as an instrument for expression, but it should also recognise when to set that instrument aside and allow for moments of meaningful silence or emptiness. In a world drowning in content, the artists of the future might be distinguished by their ability to create spaces for genuine reflection and engagement.
Memories of Passersby I is one of your well-known installations. Can you discuss the challenges in developing this project, particularly in training the neural networks to generate portraits in real-time?
As an artist who works with AI, I get questions mostly about how it works and not why I made it. You wouldn’t ask a photographer what aperture they used or a painter for the brand of their paints and brushes, would you?
Memories of Passersby I is about our expectations when faced with a screen-based, machine-driven artwork. Since I believe that there are fundamental differences in how we perceive screens and canvases – with a painting, we know that it is finished and that it will not change ever (apart from maybe some slow natural ageing processes); a screen, on the other hand, says “entertain me” since that is what we learned from years of TV-consumption or nowadays from advertising in public spaces.
So what Memories of Passersby I promises is to be ever-changing, never repeating itself, thanks to the help of a complex AI algorithm that keeps on generating new portraits out of itself. The question is whether the work meets our expectations or if we catch up to the pattern and will get bored by it after all. The “challenge” here is that the algorithm is a closed system that, after it is released into the world, will not get any new information or learn and will have to create anything new from what it already knows. In that sense, it is also a metaphor for our civilisation, which, if you look at it from very far away, has also been drawing solely from itself and its environment.
The way newness comes into this work is by using errors or accidents that happen in the internal transformation processes. In a closed feedback loop, several models are passing data between them, reinterpreting it: one model has been trained to translate a very simplified sketch of a face into an image that tries to look like a classic oil-painted portrait, the other model has been trained to reverse that process and to create a sketch of a face. Both models are imperfect in their task and, due to that, make translation errors responsible for keeping the cycle in constant change and holding the potential for the unexpected.
When I created this work in 2018, there were several technical challenges: first of all, there were no off-the-shelf face-generating models available yet, so I had first to collect my own training data set of hundreds of thousands of portraits and then train my model. During the training, I ran into what I call the “carpet problem”: imagine you have a small room and try to lay down a carpet that is too big for the space – you try to push it down on one side, but what happens is that somewhere else in the room a bubble will pop up again – you push down that bubble and it will start bulging somewhere else – all you try to do is to push the bubble in a place where you don’t notice it that much.
This is what happened with my models – their capacity to learn many different things is not unlimited. In my case, I was additionally constrained by needing access to massive GPU servers, but instead, I had to train them on my machine and the goal that the animation should run in real-time, limiting the number of layers and weights you can use. This led to the problem that, for example, the model learned to produce eyes in great detail, but the ears would just become some undefined pretzel blobs. I spent months and months experimenting with modifying model architectures or altering the training procedure until I had to settle for something that was the best I could do under the circumstances with the resources available to me.
Ironically, only half a year later, NVIDIA released the first version of StyleGAN. It made generating faces so easy and the results so much better than I could achieve that it sometimes felt like I wasted my time. I could have spent a year doing something else and waited for someone else to solve it for me. But then again, I learned so much during this process, which gave me a deep understanding of how these models work on the inside.
X Degrees of Separation is a project that delves into the connectivity between works of art. What were some significant breakthroughs and obstacles you encountered while developing this piece?
In early 2016, X Degrees of Separation was my first large commission for an artwork that employed AI. Google Arts & Culture had noticed my ongoing experimentation with classifying and digging through large image datasets—in that case, work I did with the 1 million book scans that the British Library had put into the public domain in 2013—and approached me with the opportunity to work with their huge database of cultural artefacts and the possibility of using their internal models and collaborating with their engineers.
One problem I observed with more and more cultural data becoming available for everyone to browse and search was that due to the way standard interfaces are designed, most people will rarely journey beyond the “top-100 most popular artists” or their field of interest and that 90% of the works, despite being in the open, will rarely surface. So, I proposed a different type of interface that would allow for serendipity and discovery to happen, modelled after the well-known concept of “six degrees of separation”.
The theory is that just like every human is connected to any other human on the planet through just a few linked relationships, this can also be said of artworks or cultural artefacts. Whilst the type of shared relations these works could have are typically in the metadata, like the artist who created them, the time or place of their creation or the movement out of which they came, I focussed solely on the visual aspects of the work, since that promised to me getting away from the obvious connections and the biases of art history and allow for new ways of traversing culture as well as allowing the discovery of lesser-known or documented artists without having the results distorted by popularity.
I was able to use Google’s internal feature vectors – the ones they had been using for image similarity search, which captured a lot of stylistic and semantic information about each image. Then, the principle applied is relatively simple: connect each image with several of its nearest neighbours in feature space – in practice, this means that, for example, two portraits could have a connection despite maybe one being a photograph and the other one a chalk scribble and being separated by 200 years.
Experimentally, I figured out the minimum number of neighbours so that in the end, all images would be connected through some path but not connecting each to so many that it is just one or two hops to link them. Ultimately, it needed 9 or 10 neighbours each, so no islands or isolated areas would form. This results in something that almost resembles a map with some areas that are denser populated and others that are more sparse and all connected by “roads”.
The final part is just a classic pathfinding algorithm that calculates the shortest path between any two artworks – similar to how you find the fastest route to the train station from where you are right now. At first, I implemented that algorithm myself and was quite happy when it only took 10 seconds to calculate it for a given pair. Still, fortunately, I had help from a Google engineer who optimised that code so that it eventually did the same within an eyeblink.
What’s interesting is that whilst I had imagined the final results to have some appeal, I did not know what would come out of it until it was built. And I feel that the result does precisely what I hoped it would: first of all, the “gradients” that it produces through different artforms, materials, colours or compositions often look aesthetically very pleasing and confirm the saying that the whole is greater than the sum of parts. At the same time, they can give even visually unimpressive works that only a few people would actively look for a role and a stage. And I particularly like that you can still start from two very familiar or popular works, but then on the path between them, you might discover something or someone you had never heard of.
Looking ahead, what developments or advancements in AI are you most excited about? How do you envision these changes influencing the art world?
The AI art landscape has shifted dramatically in the last decade. We’ve moved from pioneers in uncharted wilderness to interior decorators, refining what’s been established. Progress is now gradual, marked by incremental improvements rather than revolutionary leaps. Storytelling and humour remain challenging for current AI models. These domains require a nuanced understanding of context, timing, and cultural subtleties that AI must fully grasp. But I have no doubt we’ll get there, step by step.
I’ve become less excitable than I might have been ten years ago. I’m more worried than excited about certain developments – not AI progress itself, but the regulatory and political attempts to hinder it or protect the status and power of big players in this game. The most significant developments in AI art stem from how we as a society integrate and regulate (or instead not regulate) these tools rather than from technological breakthroughs. The challenge lies in keeping AI a medium for diverse artistic expression, not a tool for “surveillance creativity” controlled by a select few.
The art world will continue evolving with these developments. Interesting art might emerge not from those who use AI most proficiently but from those questioning its role, subverting it, or using it to illuminate the societal changes it’s bringing about.
What’s your chief enemy of creativity?
Time. Or rather, the forced wasting of time on tasks outside my control. This is the chief enemy of my creativity. The creative process demands uninterrupted focus, experimentation, and reflection. Yet, our world seems designed to fragment attention and fill our days with trivialities.
These impositions—bureaucratic procedures, technical troubleshooting, and the constant barrage of notifications—are creativity killers. They consume precious hours and disrupt the mental state necessary for deep work. The frustration lies in their unavoidability. They represent a cognitive tax creatives must pay to exist within society’s structures.
Ideally, artists would immerse themselves fully in their pursuits, following the natural flow of inspiration and experimentation. Instead, we constantly negotiate with time, trying to carve out spaces for creativity amidst a sea of obligations. This battle against time-wasting is one of the most significant yet least discussed challenges for artists in the digital age. As our tools grow more powerful and our creative potential expands, protecting and maximising our creative time becomes increasingly crucial.
You couldn’t live without…
…my most significant other, Alexandra, my dog Mabel, coffee, cigarettes and solitude.