Text by CLOT Magazine
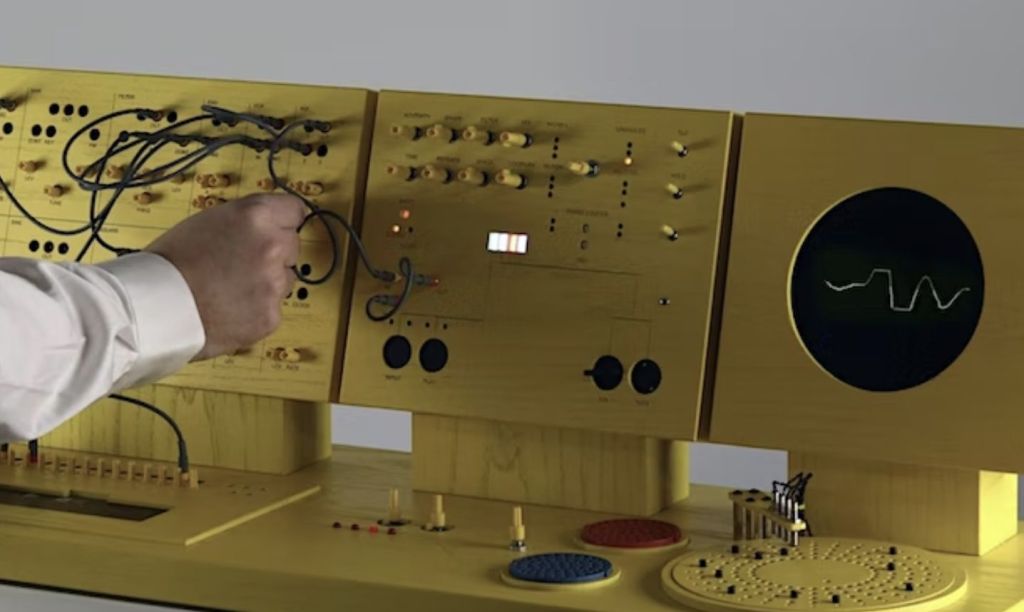
Dr Rebecca Fiebrink is a Professor of Creative Computing at the UAL Creative Computing Institute in London. Inventor of the Wekinator, a tool that has contributed significantly to the proliferation and democratisation of AI in the creative space, her research focuses on designing new ways for humans to interact with computers in creative practice, including using machine learning as a creative tool: My own software enables people to build completely new digital musical instruments by demonstrating the ways they want to move when they play alongside the sounds they want to result from those movements: the machine learning here infers the creator’s desired relationships between movement and sound, and reproduces them in the new instrument.
Machine learning models can be used to uncover the patterns that underlie music of beloved artists or genres, opening up new opportunities to build new immersive experiences, learn from the patterns that exist in musical and non-musical real-world sounds, and produce variations on these in order to synthesise totally new sounds nobody has heard before. The opportunities for supporting human creation and engagement are likely the areas for an impact that really make a difference to most people.
The Wekinator attempts to encourage creativity by making it possible for anyone with a computer to create new real-time interactions with digital processes. Originally, this meant enabling anyone to create new digital musical instruments by creating new linkages from a performer’s movement to computer-synthesised sounds. Dr Fiebrink explains over Email.
But in the last 13 years, this has expanded to include all sorts of other interactions in which one or more people’s (or animals’) actions drive changes in music or sound processes, live animations and video, lighting, game engines, smart home automations, Google Maps locations, or other things. The barriers to people creating and experimenting with AI are frequently due to cutting-edge AI systems requiring a lot of programming and machine learning expertise and access to expensive hardware. However, the key bits here are that people don’t need to know much about machine learning or programming to use it and that you build an interaction from examples of what might happen in the world and what the computer might do in response rather than by writing code.
Dr Fiebrink told us that one of the consequences for creativity when using the Wekinator is that it’s easy to access serendipitous moments in which the machine learning does something pleasantly surprising — different from what you expected but still in the realm of something that might work, and this can also be enjoyable and lead people in new creative directions.
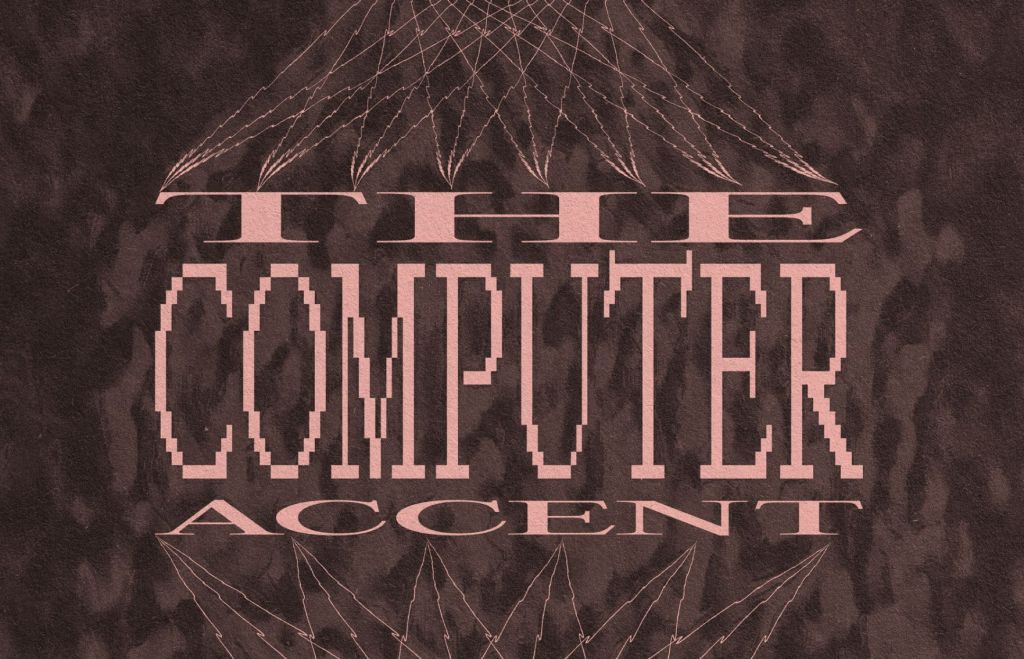
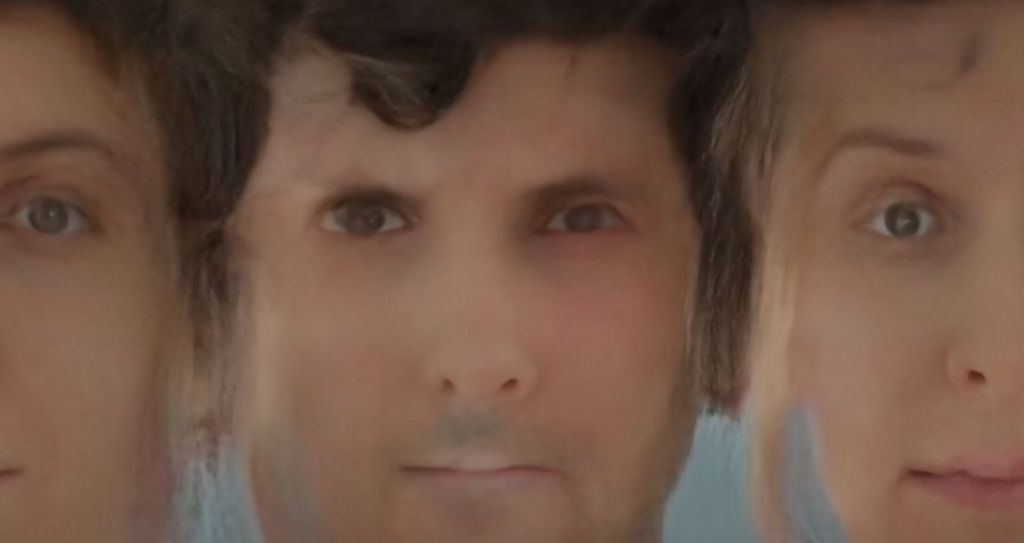
In the last years, there has been an explosion of interest in machine learning algorithms and AI capable of creating new images, sound, and other media content. Some examples highly known that have impacted the landscape of creation are, for example, Jennifer Walshe’s and Memo Akten’s ULTRACHUNK work, which involves performing live on stage with one’s own musical “doppelgänger,” and Holly Herndon’s deep-fake model of her own voice, which she’s released to the public for anyone to use.
But how is AI-generated art/music changing the paradigms of art/music? I don’t think that AI is necessarily changing the paradigms of art or music, in the sense that I don’t necessarily see our definitions of what art or music are changing substantially. However, I think AI is changing the precise types of activities we can engage in when being creative. These kinds of changes can improve what novices can accomplish when making work that fits into existing paradigms and enable experts to work more effectively or quickly. Dr Fiebrink points out.
That the machine learning models are notoriously full of biases is not new for any of us. This has received a lot of attention when models incorporate and reproduce societal biases in obviously harmful ways, for instance, when a language generation model produces racist, sexist hate speech. Gabriel Vigliensoni, one of Dr Fiebrink’s postdoc students, has shown that other types of bias are important when we’re using generative machine learning models for creative work. Dr Fiebrink told us that Gabriel’s work has further shown that the representations we use when we’re working with symbolic data (like MIDI or musical scores) rather than audio recordings themselves can be even more biased and limiting. Most existing rhythm models that work from symbolic representations only allow simple meter, which means that a musical beat can only be divided into 2, 4, or 8, etc. It can’t be divided into 3. This makes it impossible to even represent rhythms from much music from Latin America or Africa and some modern electronic dance music. Gabriel’s research has therefore had to involve training entirely new rhythm models that use different representations capable of handling more complex rhythms.
If you would like to learn more about machine learning and AI for creative purposes this Wednesday at 7:00 pm at HQI, the Rotunda, White City, London, Dr Rebecca Fiebrink is running a workshop on music and the role of machine learning/AI. She hopes that people will leave with a better understanding of where AI may take creative work and potentially some links to open-source tools they could enjoy using again.
Find tickets here.