Interview by Bilge Hasdemir
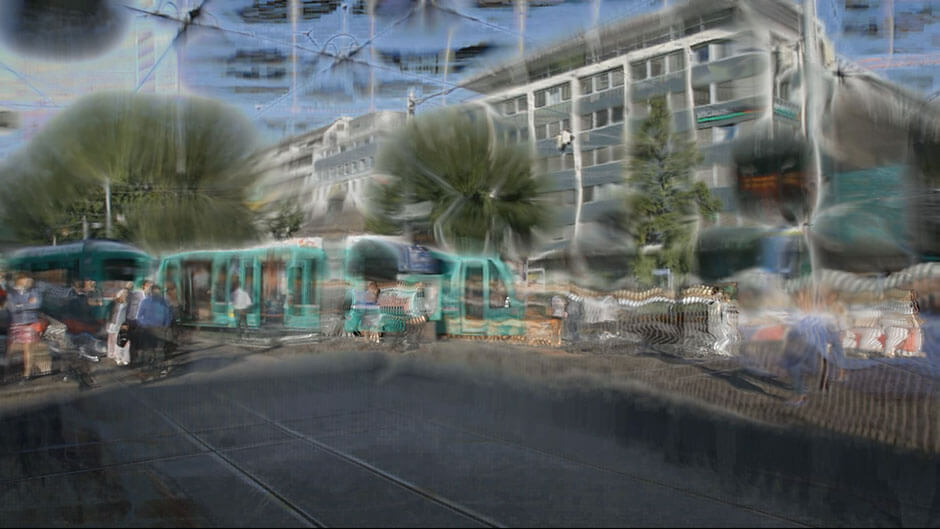
Ursula Damm is a media artist working at the intersection of art, science and technology on a delicately balanced ground. Besides, she is a sculptor as well. Since 2008 Damm holds the chair of Design of Media Environments at Bauhaus-University Weimar, where she is currently working as a professor and is also involved in establishing the Digital Bauhaus Lab and DIY BioLab. Damm’s work often engages with system interactions on different grounds. In that vein, machine learning, genetic algorithms, neural networks, and also synthetic biology are just some of the topics she has been researching up until now.
She has been expanding the viewer’s field of experience by using computational methodologies inspired by nature since she explored possibilities of biological processes to go beyond human thinking. By challenging anthropocentric understandings, she looks at natural systems and their communication strategies to determine how an artist can work on a livable future.
Her ways of approaching inter-connections and cooperations in different systems have inspirations from Jacob von Uexküll’s notion of Umwelt (German for “environment”), which has influenced several fields of studies like biosemiotics and cybernetics.
Through her art, Damm invites us not only to think about the existence of many other forms of life(s) other than our own but also to overcome our own human-centred ‘Umwelt’ to establish shared environments. Lately, she has been working on machine learning which enables the viewer to experience the “imagination” of a computer by revealing the machine’s way of capturing, interpreting and manipulating visual input.
Membrane is one of her recent projects based on the TGAN algorithm (Temporal Generative Adversarial Nets), which will generate numerous possibilities to perform dynamic learning on both short and long timescales and ultimately be controlled by the user/ visitor. By bringing autonomy to the algorithm on the basis of its self-organized temporality, the algorithm is radicalised, and the capacities of machine learning are extended.
Transits, presented at Fort Process, traces of the passersby at Aeschenplatz in Basel filmed during a 24-hour period. By integrating an artificial neural network into the software in use, the video footage of crossing movements was processed in accordance with a self-organised way through which the image slowly became a painting. With Transits, Damm enhances visitors’ perception of the city within a multifaceted experience by adjusting the information emerging from the city to the human brain’s capabilities.
Adding a new layer in Chromatographic Ballads by means of an EEG device, Damm allows visitors to navigate with her/his brain waves and to define the degree of abstraction of a generative algorithm.
Through her art, Damm closes the gap between two cultures: art and science. Without a doubt, Damm is one of the significant names in a Third Culture that enables us to explore the thought-provoking potentials of the convergence of art and science at a joint presence. In addition, many of her works critically draw from the cultural impact of the day’s technologies, which also follows a question on our relationship with technology.
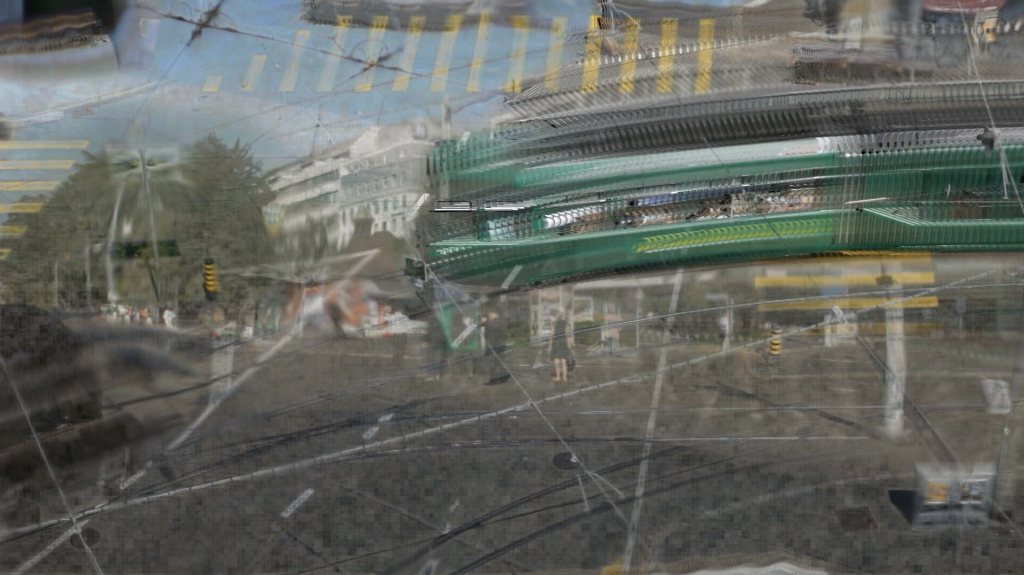
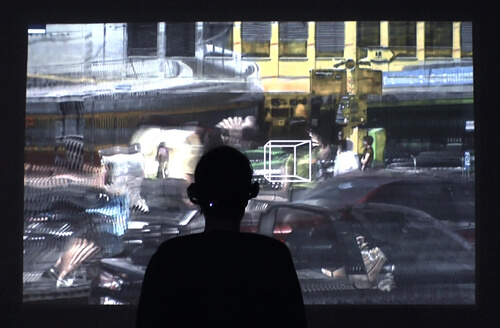
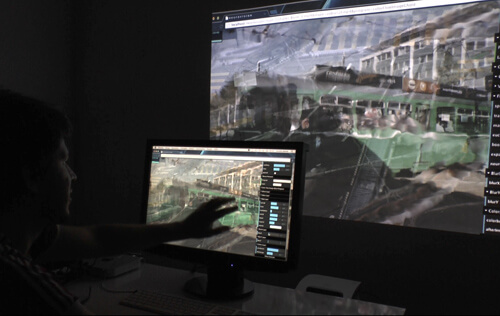
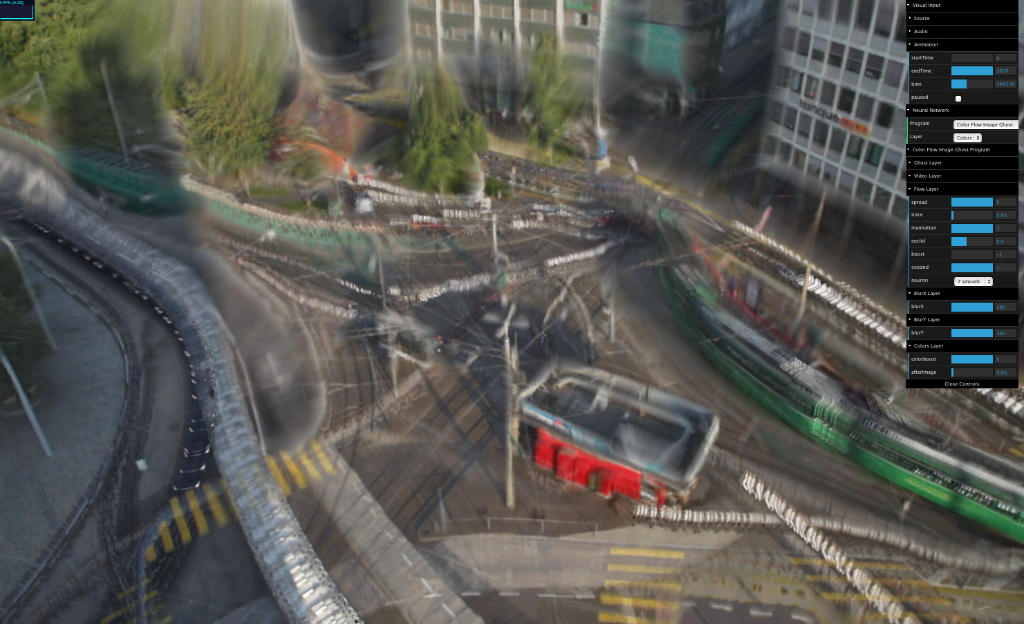
Your artistic practice involves working and researching system interactions. Using new media, you scrutinise aspects of the social interaction of human and animal communities and their ecosystems while exploring their interaction with computer science (more recently, machine learning and neural networks). And with his bringing your art to a technological context. How and when the interest in these different disciplines comes about?
During my studies at the KHM Cologne, I learned that information science uses biological strategies to obtain knowledge beyond human thinking. Concepts like neural networks and genetic algorithms were subjects of the teaching of Georg Trogemann, Professor of Experimental Informatics. At this time, I was fascinated by the possibility of optimising the behaviour of virtual agents with evolutionary algorithms (double helix swing) or analysing datasets with self-organising maps (memory of space or other works with neural networks).
Soon it became obvious that we humans are still at the beginning of understanding evolution, mind or consciousness. Nature holds far better concepts. This is why I started using biological concepts of computing and feedback to situate my works within a larger (biological) environment. In 2009, a co-worker and lecturer, returning from the RCA to Weimar, called it old-fashioned to use a classical computer for computation.
He claimed that we would calculate in the future ‘in the living’ within the genetic code of organisms and cells, as it is done in Synthetic Biology. Triggered by my experiences with genetic algorithms, he convinced me to start a collaboration with Bioquant, a research centre at Heidelberg University, for an interdisciplinary IGEM Team1.
Convinced that information technology and biotechnology is altering our culture faster than any art movement we could imagine, it became necessary to develop a strategy for how artists can work on a liveable future. This is why I look at natural systems and their communication strategies. How did evolution move forward?
Can our technologies be a surplus value not only for an efficient economy but also for our culture? What is the cultural impact of our technologies? And how do we want to plan them before this background? It was the biosemiotics of Jakob von Uexküll delivering a theory on communication, interaction and world-making – a long time before we spoke about networks and communication theory.
Through the concept of ‘Umwelt’, he laid the foundations for understanding the construction of an own internal “world” of a living being through its interaction with its environment. Identifying an objective region of the stimulus sphere allowed the description of a model of memory and action between the individual and its surroundings. Using such a construct, we adopted Uexküll’s respect for the completeness of the individual world (what he names ‘Umwelt’) and his understanding of the effects of stimuli in the self-understanding of a being2.
Uexküll developed tools and interfaces for animals (page 30). Some tools were made to investigate the world’s making of snails. Others were made to help dogs to understand the ‘Umwelt’ of humans3. Uexküll inspired us to build interfaces for humans and animals, allowing us to experience the ‘Umwelt’ of the mutual other. By doing so, we are establishing new forms of technology-enhanced feedback systems and developing the aesthetics of exchange and communication across species. Thus we hope to overcome our own human-centred ‘Umwelt’ to establish shared environments.
One of your most recent projects, Membrane, is an installation specifically on machine learning, TGAN Algorithm. What is unique about this piece?
Membrane is an art installation which is planned to be exhibited in Berlin next spring. It builds on a series of generative video installations with real-time video input. Membrane allows the viewer to interact directly with the generation of the image. In doing this, it is possible to experience the computer’s imagination, guiding the process according to curiosity and personal preferences.
An image on a computer is represented by a matrix of RGB values along an x- and a y-axis. A digital image consists of a grid of pixels which take their values from a spectrum of hues (usually 256 per channel). In the case of Membrane, these images are derived from a static video camera observing a street scene in Berlin. In this respect, we can view the image as a snapshot of the place within a certain time frame. Thus we are able to interfere with the temporal alterations of the image on an algorithmic level via software.
Generally, self-organised neural networks make reductions to the complexity of a set of data which, at first glance, looks like a video filter. The possible interpretations of an image are systematically reduced until you can make a statement about the content of the image (for example: yes, it’s a dog, or no, there is no dog). Neural Networks, a form of machine learning, work with interconnected sensors that can store information and have perceptive capabilities.
These so-called neurons measure and judge packets of data which are channelled through them whilst simultaneously adapting to the processed data. Reciprocal feedback is established, which operates without the use of external categories.
A neuron is defined solely by the network architecture (i.e. the connections between the neurons). The set of neurons generates an abstract, netlike learning environment which can (re)produce datasets with specific properties and evaluate resemblances or distinctions. Humans perceive these procedures as ‘subsymbolic’, working without labels, symbols or metaphors.
In my earlier artistic experiments within this context (Transits, 2012), we considered each pixel of a video data stream as an operational unit. One pixel learns from colour fragments during the programme’s running time and delivers a colour which can be considered as the sum of all colours during the running time of the camera.
This simple memory method creates something fundamentally new: a recording of movement patterns at a certain location4. Here, it becomes obvious how the arrangement of input devices, processing and output is able to create new worlds and contexts.
On a technical level, Membrane controls pixels or clear-cut details of an image and image ‘features’ which are learnt, remembered and reassembled. Regarding the example of colour: we choose features, but their characteristics are delegated to an algorithm. TGANs (Temporal Generative Adversarial Nets) implement ‘unsupervised learning’ through the opposing feedback effect of two subnetworks: a generator produces short sequences of images, and a discriminator evaluates the artificially produced footage.
The algorithm has been specifically designed to produce representations of uncategorised video data and – with its help of it – to produce new image sequences5. We extend the TGAN algorithm by adding a wavelet analysis which allows us to interact with image features instead of only pixels from the start. Thus, our algorithm allows us to ‘invent’ images more radically than classical machine learning would allow.
In practical terms, the algorithm speculates on the basis of its learning and develops its own self-organised temporality. However, this does not happen without an element of control: feature classes from a selected data set of videos are chosen as target values. The dataset consists of footage from street views of other cities worldwide, taken while travelling.
The concept behind this strategy is not to adapt our visual experience in Berlin to global urban aesthetics but rather to fathom the specificity and invent by associations. These associations can be localised, varied and manipulated within the reference dataset. Furthermore, our modified TGAN algorithm will generate numerous possibilities to perform dynamic learning on both short and long timescales and ultimately be controlled by the user/ visitor.
The installation itself allows the manipulation of video footage from an unchanged street view to a purely abstract image based on the found features of the footage. The artwork wants to answer the question of how we want to alter realistic depictions. What are the distortions of ‘reality’ we are drawn to? Which fictions are lying behind these ‘aberrations’? Which aspects of the seen do we neglect? Where do we go with such shifts in image content, and what will be the perceived experience at the centre of artistic expression?
The fictional potential of machine learning has become popular through Google’s deep-dream algorithms. Trained networks synthesise images on the basis of words and terminologies. They are reconfiguring symbols which they allegedly deem to recognise.
From an aesthetic perspective, these images look paranoid; instead of presenting a consistent approach, they tail off in formal details and reproduce previously found artefacts (through searching the internet). From an artistic point of view, the question now arises, how can something original and new be created with algorithms? This is the question behind the software design of Membrane.
Unlike Google’s deep-dream algorithms and images, we don’t want to identify something specific within the video footage (like people or cars). Still, rather we are interested in how people perceive the scenes. That is why our machines look at smaller, formal image elements and features whose intrinsic values we want to reveal and strengthen. We want to expose the visitors to intentionally vague features: edges, lines, colours, geometrical primitives, and movement.
Here, instead of imitating a human way of seeing and understanding, we reveal the machine’s way to capture, interpret and manipulate visual input. Interestingly, the resulting images resemble pictorial developments of classical modernism (progressing abstraction on the basis of formal aspects) and repeat artistic styles like Pointillism, Cubism and Tachism in a uniquely unintentional way.
These styles fragmented the perceived as part of the pictorial transformation into individual sensory impressions. Motifs are now becoming features of previously processed items and successively lose their relation to reality. At the same time, we question whether these fragmentations of cognition are proceeding arbitrarily or whether other concepts of abstraction and imagery may be ahead of us.
From a cultural perspective, two questions remain 1) How can one take decisions within those aesthetic areas of action (parameter spaces)? and 2) Can the shift of the perspective from analysis to fiction help to asses our analytical procedures differently – understanding them as normative examples of our societal fiction serving predominantly as a self-reinforcement of present structures?
Thus unbiased artistic navigation within the excess/surplus of normative options of actions might become a warrantor for novelty and the unseen.
In Transits, the piece you are presenting at Fort Process, you developed a neural network forming a complex system that interprets the daily movement in a city (cars, public transport people, and translates it into a visual flow. What was the intellectual process behind this project?
Transits deals with video footage of a crossing (here: the Aescheplatz at Basel, Switzerland) as if it would be a painting. I first choose a motif of interest: a crossing within a city with multiple transport users: cars, tramways, pedestrians – mingled in a specific time-space pattern. This pattern works very well with the algorithm carving out the traces of the moving subject.
In addition to simple movement traces, we allowed the algorithm to behave self-organised: to grow colour areas and push neighbouring pixels into the direction of an apparent motion. Thus, the image slowly becomes a painting resembling Ernst Wilhelm Nay’s tableaux rather than a bustling crossing video. Martin Schneider developed the Neurovision framework – a software mimicking the human brain’s visual system. Thus we question whether our algorithms meet the spectator’s well-being by anticipating our brain’s processing steps.
With this software, we are questioning how much complexity our senses can endure. Or rather, how could we make endurable what we see and hear? Many communication tools have been developed to adjust human capabilities to the requirements of the ever more complex city. Our installation poses the opposite question: How can information emerging from the city be adjusted to the capabilities of the human brain? Hence, processing them is a pleasure to the eye and the mind.
We critically assess the effect of our installation on the human sensory system: 1) Does it enhance our perception of the city meaningfully? 2) Can it, and if so – how will it affect the semantic level of visual experience? 3) Will it create a symbiotic feedback loop with the visitor’s personal way of interpreting a scene 4) Will it enable alternate states of consciousness? 5) Could visitors experience the site in a sub-conscious state of “computer-augmented clairvoyance”?
After finishing Tansits (which had been only exhibited as a generative video), Martin Schneider and I wished to let the software perform: We connected Neurovision to a live camera and created an interface for an operator with an EEG headset.
Thus ‘Chromatographic ballads’ allows a visitor to navigate within the parameter space of the respective neural layers of the software to experience the image space of the installation unconsciously. Although the installation was only shown in a private experimental setting, it obtained an honorary mention at Vida 15.0 in 2013. With Membrane, we hope to finally make a software framework accessible and tangible to a larger audience.
Your work with neural networks and artificial intelligence involves working intimately with scientists. What are the most significant challenges that both (yourself and the scientist’s teams) faced in the development of these projects?
For an artist, the most challenging aspect is that you need to plan your work on a formal, technical level in a foreign language. I did not yet grow up with training in informatics. I learned to program at the age of 35. My artistic approach was formed by sculptural aspects and conceptual thinking and questions related to installation art. These domains are clearly separated from a scientific approach (‘two cultures’) within our cultural sphere.
The problems become easily obvious once you plan a software. Before you start to work, you have a sensual imagination about your future artwork. But during the development, you cannot change the overall concept of your doing to adapt it to your senses (as you can do in traditional art practices). The outcome is anticipated in your plans.
This kind of working practice requires a lot of experience to allow ‘artistic degrees of freedom’ in your software. This is why you urgently need skills in programming and informatics to be able to meaningful elaborate the potential of ‘algorithmic fiction’ (I will soon publish an article on that together with Georg Trogemann).
However, not only the conceptual aspects are challenging. Many of my high-level science collaborations ended as we could not find any financial support for common projects. Our art is becoming increasingly specialised and requires competent scientists to cooperate. But our art system does not provide the budgets to employ regular scientists or to work in a way allowing us to communicate with scientists on an eye level. And scientists are not able to share their budgets with artists.
On the other hand, many scientists are reluctant to cooperate as many popular art & science projects are fakes and scientifically untenable. We need formats allowing artists to get seriously involved with scientific methods, and we must convince our audience that we cannot explain everything in 10-20 minutes. We need more long-range grant opportunities for interdisciplinary artistic projects!
You also have a long academic trajectory, and since 2008 you hold the chair for Media Environments at the Bauhaus University in Weimar, where you are also involved in establishing the Digital Bauhaus Lab. Are there still any unexplored territories you would like to take your research into?
In Weimar, we established a motion capture and VR environment and a DIY Biolab. Both places are full of potential and not yet fully explored. My initial goal was to design interactive architecture (similar to Fernfuehlers). My experiences during the last years show that it is appropriate to let the works grow with your practice, step by step. One challenge would be to explore the impact of VR on the bodily and cognitive construction of identity. In our Biolab, I will work with growing city plans.
What is your chief enemy of creativity?
The ‘normal’ – and being tied to predictions out of the past without any chance to experience the unforeseen.
You couldn’t live without…
Music.